The rise of artificial intelligence (AI) and machine learning (ML) is transforming multiple industries, and digital forensics is no exception. Investigators now leverage advanced AI algorithms to analyze vast amounts of digital evidence, detect anomalies, and uncover patterns that human analysts might miss. As the digital landscape grows increasingly complex, AI-driven digital forensic services are proving to be indispensable tools for law enforcement, legal professionals, and private investigators.
The Role of AI in Digital Forensics
Digital forensics involves collecting, analyzing, and interpreting electronic data to uncover digital crimes. Traditionally, this process required extensive manual effort and could take weeks or months to yield results. AI, particularly machine learning, accelerates this process by automating data sorting, identifying suspicious activity, and assisting in expert witness testimony with data-backed insights. AI’s ability to learn from past cases also enhances the accuracy and reliability of forensic investigations.
Machine Learning in Data Analysis
One of the most significant benefits of AI in digital forensics is its ability to process and analyze large datasets quickly. Machine learning algorithms sift through emails, messages, logs, and multimedia files to identify patterns indicative of cybercrime, fraud, or data breaches. AI tools can highlight correlations that may not be immediately apparent to human investigators, enabling faster and more precise decision-making.
AI-Powered Pattern Recognition
Pattern recognition is a cornerstone of modern forensic investigations, allowing experts to detect anomalies and uncover hidden connections within vast datasets. AI-driven pattern recognition enhances this process by leveraging machine learning algorithms trained on extensive cybercrime datasets. These models can analyze digital communications, financial transactions, and network traffic to identify irregular behaviors that might indicate illicit activities.
For instance, AI can detect subtle changes in messaging patterns, such as sudden spikes in encrypted communication or deviations from a user’s typical writing style, which may signal insider threats or phishing attempts. In financial transactions, AI can flag suspicious transfers that resemble money laundering schemes by recognizing patterns across multiple accounts, jurisdictions, or currencies. Similarly, in network traffic analysis, AI-powered tools can monitor real-time data flows, identifying anomalies such as unauthorized access attempts, unusual data transfers, or coordinated cyberattacks.
Predictive Analysis and Anomaly Detection
Machine learning algorithms can also predict future cyber threats by analyzing historical data and identifying emerging attack vectors. Anomaly detection techniques allow forensic analysts to recognize deviations from normal digital behaviors, enabling proactive security measures. This predictive capability is crucial for preventing cybercrimes before they escalate.
Audio Authentication Services and AI
With the increasing prevalence of voice recordings as digital evidence, AI-driven audio authentication services have become a vital tool in forensic investigations. Advanced machine learning models analyze audio files to detect signs of tampering, identify speakers, and verify authenticity.
Voice Biometrics in Digital Forensics
Voice biometrics leverage AI to create unique voice signatures based on speech patterns, pitch, and tone. These biometric markers help forensic analysts match recordings to specific individuals, adding credibility to expert witness testimony in court. AI-driven voice recognition enhances the accuracy and reliability of speaker identification, which is particularly useful in fraud investigations and legal disputes.
Deepfake Detection: Combating Manipulated Audio Evidence
As deepfake technology advances, so does the risk of manipulated audio being used as false evidence in legal proceedings, fraud schemes, and misinformation campaigns. Deepfake audio, generated using sophisticated AI models, can mimic real voices with remarkable accuracy, making it difficult for the human ear to distinguish between authentic and fake recordings. This poses a significant threat to digital forensics, as falsified voice recordings can be used to impersonate individuals, fabricate confessions, or manipulate financial transactions.
To counter this, AI-powered forensic tools leverage advanced machine learning algorithms to analyze multiple characteristics of an audio file. These tools scrutinize speech anomalies, such as irregular pacing, mismatched phonemes, and unnatural intonations, which may indicate AI synthesis rather than genuine human speech. Additionally, forensic AI examines background noise inconsistencies, identifying whether ambient sounds align naturally with the environment in which the recording was allegedly made. Mismatched reverberation, sudden audio drop-offs, or inconsistencies in microphone quality can signal tampering.
Another key factor in deepfake detection is voice modulation analysis. While deepfake models can replicate tone and pronunciation, they often struggle with dynamic variations in speech, such as emotional fluctuations, subtle stress patterns, or natural hesitations. AI forensic tools use frequency analysis and spectrogram comparisons to detect these unnatural modulations.
Image and Video Analysis in Digital Forensic Services
Another area where AI is revolutionizing digital forensics is in image and video analysis. Machine learning models trained on forensic datasets can detect forged images, identify manipulated video content, and extract critical metadata that might otherwise be overlooked.
- Object and Facial Recognition
AI-based digital forensic services employ facial recognition algorithms to match suspects to footage captured from security cameras, social media, or mobile devices. These tools help investigators verify identities, track movements, and gather evidence for criminal cases. AI-powered object recognition can also identify specific items within images or videos, aiding in criminal investigations and court proceedings.
- Scene Reconstruction
Machine learning enables digital forensic experts to reconstruct crime scenes from fragmented data, such as partially deleted images or low-quality surveillance footage. AI-enhanced video enhancement techniques clarify blurred or pixelated visuals, improving the quality of digital evidence.
AI in Mobile Forensics
Mobile devices are central to modern digital investigations. AI helps forensic experts extract, analyze, and interpret data from smartphones, tablets, and other digital devices efficiently.
- Automated Data Recovery
AI-powered tools can recover deleted messages, emails, call logs, and app data from mobile devices. This is particularly useful in criminal investigations, where deleted evidence can hold critical clues. AI algorithms scan through file remnants to reconstruct lost or corrupted information.
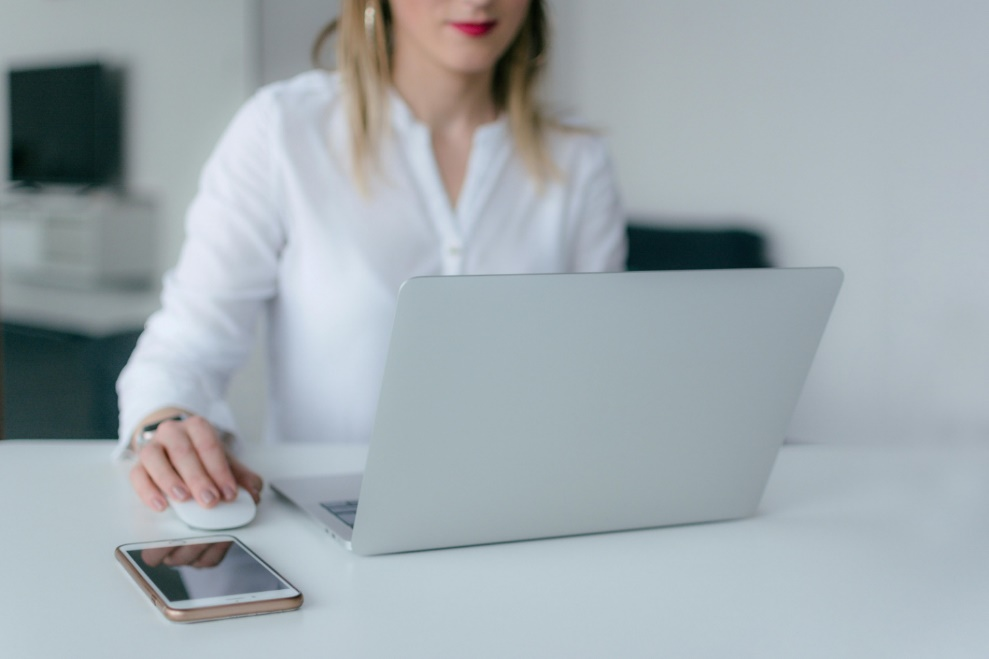
- Geolocation and Behavioral Analysis
Machine learning models analyze geolocation data to establish movement patterns and identify locations of interest in an investigation. Behavioral analysis can also detect irregular mobile activity, such as unauthorized access to sensitive files or abnormal device usage.
AI and Expert Witness Testimony
Expert witness testimony is a crucial component of legal proceedings, and AI enhances the credibility and precision of forensic evidence presented in court.
- AI-Backed Reports and Analysis
AI-powered forensic tools generate detailed, data-driven reports that help expert witnesses provide clear, factual explanations of digital evidence. These reports include visualizations, statistical analyses, and forensic timelines that strengthen the prosecution or defense’s argument.
- Ensuring Unbiased Testimony
Machine learning models operate on data-driven principles, reducing the risk of human bias in forensic investigations. AI-generated insights are based purely on factual data, ensuring that expert witness testimony remains objective and reliable.
AI in Malware and Phishing Detection
AI algorithms play a critical role in modern cybersecurity by identifying and mitigating threats such as malware infections and phishing attacks. Traditional antivirus programs rely on signature-based detection, which requires a known database of threats to function effectively. However, AI-powered systems go beyond these limitations by leveraging behavioral analysis, anomaly detection, and real-time threat intelligence.
Machine learning models analyze vast datasets from previous cyberattacks, recognizing subtle patterns in malicious email content, suspicious URLs, and unauthorized software behavior. For example, AI can scan incoming emails for phishing indicators such as deceptive domain names, mismatched sender addresses, and unusual language patterns. By using natural language processing (NLP), AI can differentiate between legitimate and fraudulent messages, reducing the risk of employees falling victim to phishing scams.
In malware detection, AI employs deep learning techniques to assess the behavior of software applications. Unlike traditional methods that rely on predefined signatures, AI-driven malware detection identifies new and evolving threats by monitoring how programs interact with system resources. This proactive approach allows security teams to detect zero-day attacks—previously unknown vulnerabilities exploited by hackers.
Furthermore, AI-powered forensic tools assist investigators in tracing cybercriminal activities. By analyzing network traffic, AI can uncover hidden command-and-control (C2) communications, revealing the infrastructure behind large-scale cyberattacks. These insights help law enforcement agencies track down cyber criminals, disrupt botnets, and prevent further breaches.
As cyber threats become increasingly sophisticated, AI continues to evolve, improving its ability to detect and respond to emerging dangers. The integration of AI in cybersecurity strengthens digital forensic capabilities, enabling faster, more accurate investigations and enhancing overall digital security.
Blockchain Forensics
AI also assists in blockchain forensics by analyzing cryptocurrency transactions to track illicit financial activities, such as money laundering or fraud. AI tools can identify suspicious transaction patterns and trace funds across multiple digital wallets.
Ethical Considerations and Challenges
While AI provides significant advancements in digital forensics, it also raises ethical concerns and technical challenges.
Privacy and Data Protection
The use of AI in forensic investigations must balance law enforcement needs with privacy rights. Regulations such as GDPR and other data protection laws require forensic experts to handle digital evidence responsibly.
AI Bias and False Positives
AI models in digital forensics rely heavily on the quality and diversity of the data they are trained on. If the training data is biased—whether due to underrepresentation of certain demographics, incorrect labeling, or systemic flaws—AI can produce skewed results. This bias can manifest in various ways, such as misidentifying individuals in facial recognition software, flagging innocent behaviors as suspicious, or generating false positives in cybercrime detection.
False positives are particularly problematic in forensic investigations because they can lead to wrongful accusations, wasted resources, and legal challenges. For example, an AI system designed to detect anomalies in network traffic may mistakenly flag legitimate activity as a cyberattack, causing unnecessary investigations and delays. Similarly, an AI-powered audio authentication service might misinterpret background noise distortions as evidence of tampering, leading to incorrect conclusions about the authenticity of a recording.
To mitigate these risks, forensic AI systems require continuous refinement, including rigorous testing on diverse datasets, regular updates to account for new patterns, and human oversight in decision-making. Transparency in AI decision-making processes—such as explainable AI (XAI)—is crucial to ensuring that forensic experts and legal professionals understand how conclusions were reached. By addressing bias and reducing false positives, AI can enhance the credibility of digital forensic services and strengthen the reliability of expert witness testimony in legal proceedings.
Legal Admissibility of AI-Generated Evidence
Courts require strict verification of AI-generated forensic evidence before accepting it as valid. Forensic experts must be able to explain AI methodologies in a manner that judges, jurors, and legal professionals can understand.
AI and machine learning are revolutionizing digital forensics by enhancing data analysis, improving evidence authentication, and supporting expert witness testimony. However, ethical considerations and legal challenges must be addressed to ensure responsible and effective use of AI in digital investigations.
Need reliable digital forensic services for your case? At Eclipse Forensics, they use advanced AI and audio authentication services to analyze digital evidence with precision. Whether you need deepfake detection, voice verification, or expert analysis, their team delivers trusted results. They also provide expert witness testimony to support your legal proceedings. Contact them today!